Introduction to Large Language Models (LLMs)
Unlocking the secrets of Large Language Models (LLMs) could hold the key in ensuring elon
musk like productivity in 2024. This cutting-edge subset of pretrained foundational models
are revolutionizing the way we think and create. Whether you are a writer, researcher,
entrepreneur or technologist looking to automate mundane tasks, LLMs should be on your
everyday tool list. In this blog, we'll dive into the fascinating world of LLMs and discover how
they are able to increase your productivity by up to 5X!
But First, What are LLM’s
LLMs, or Large Language Models, are advanced artificial intelligence models designed for
natural language understanding and generation. They leverage deep learning techniques,
particularly transformer architectures, to process and generate human-like text. Examples
include GPT-3 (Generative Pre-trained Transformer 3) developed by OpenAI.

What are the Benefits of Using LLMs?
Large Language Models (LLMs) simplify content generation, offering instant and accurate
assistance, for tasks like email drafting. They aid brainstorming by providing diverse
responses, fostering new insights.
Best thing is LLMs are versatile tools applicable in various industries, streamlining processes
from content creation to customer support, and excel in language translation with
multilingual capabilities. The use cases are evergrowing.
In this blog however, We’re going to look at how large language models saves time via
automating repetitive tasks. Freeing up treasured resources in your organization to address
more strategic tasks.
But here’s a brief summarization of the potential benefits, and some popular LLM’s in the
market.
Why use LLM’s
- Efficiency in Content Creation
- Improved Writing Assistance
- Enhanced Communication
- Code Generation and Assistance
- Language Translation
- Research Support
- Task Automation
- Learning and Skill Development
- Personalized Productivity Tools
- Innovation in Business Processes
It's important to note that while LLMs offer numerous benefits, users should be mindful of
ethical considerations, data privacy, potential biases and errors. Additionally, depending on
the specific application, some tasks may still require human oversight and intervention.
Examples of Popular LLMs
There are several Large Language Models (LLMs) that have received tremendous attention
in current years. One such example is OpenAI's GPT-3, which stands for Generative Preeducated Transformer 3. This powerful version has a whopping a hundred seventy-five
billion parameters, permitting it to generate relatively sophisticated and contextually
relevant text.
Other popular LLMs include:
1. Turing NLG: Microsoft's Turing NLG, capable of knowledge human language at a
remarkable stage. It can provide correct solutions to complex questions or even
create coherent memories when given only a few activates.
2. Meena : Google has its very own LLM referred to as Meena, designed especially
for carrying out open-ended conversations. With its vast know-how base and natural
language processing abilities, Meena can keep meaningful discussions on diverse
subjects.
3. PaLM: Google's Pathways Language Model (PaLM) is a transformer language
model capable of common-sense and arithmetic reasoning, joke explanation, code
generation, and translation.
4. BERT: The Bidirectional Encoder Representations from Transformers (BERT)
language model was also developed at Google. It is a transformer-based model that
can understand natural language and answer questions.
5. XLNet: A permutation language model, XLNet generated output predictions in a
random order, which distinguishes it from BERT. It assesses the pattern of tokens
encoded and then predicts tokens in random order, instead of a sequential order.
6. BlenderBot : Facebook's Blender bot is any other famous preference among
builders. It excels in conversational AI and has been skilled on a diverse variety of net
data to address one-of-a-kind verbal exchange styles comfortably.

These examples demonstrate the tremendous capacity of LLM generation across numerous
domains which include content creation, customer support chatbots, digital assistants, and
more.
How to Incorporate LLMs into Your Workflow
Incorporating Large Language Models (LLMs) into your workflow is vital and here are some
guidelines to leverage LLMs in your everyday routine.
1. Familiarize yourself: Take the time to explore and recognize the benefits that
LLMs offer. Whether it is generating content material, answering questions, or
providing pointers, knowing what your preferred will do wonders
2. Identify unique use cases: Determine which tasks will benefit you the Whether
you need assistance with writing articles, drafting emails, or even brainstorming
thoughts, having a clear idea of how you intend to make use will streamline your
workflow.
3. Customize and exceptional-track: Many LLMs permit for personalisation with the
aid of schooling them on particular datasets applicable in your area or enterprise. By
tailoring the model's understanding base to align along with your needs, you can
improve its overall performance and accuracy for specialised tasks.
4. Integrate with existing technology: Look for methods to seamlessly combine LLMs
into the data and infrastructure investments that are already shape part of your
environment.
5. Practice green querying techniques: When interacting with an LLM via prompts or
queries, recall that being unique in what you ask yields better outcomes. Experiment
with exceptional phrasing techniques and study from the responses received until
you discover an approach that always gives you correct outputs.
Did you know:-
Prompting is the act of instructing a language model with input to generate desired outputs. A
good prompt is clear and specific, like "Write a poem about nature," while a bad prompt is
vague, such as "Write something." Clear prompts yield coherent and relevant responses,
while ambiguous ones may result in unpredictable or nonsensical outputs.
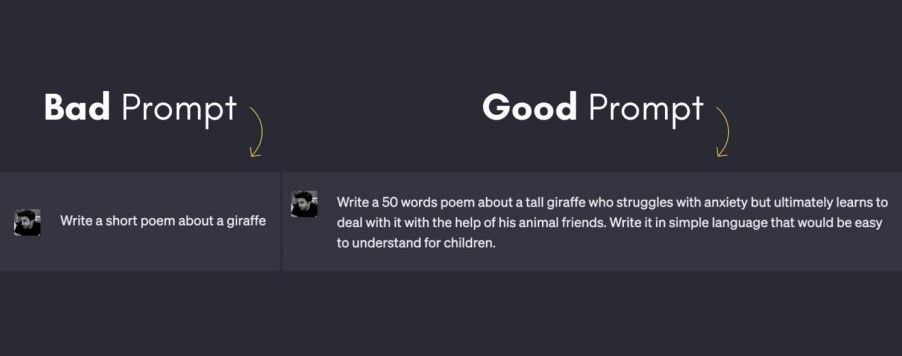
Source: Geekflare
Making it Fun and Structured
Collaborate with Colleagues: Share insights, talk outcomes, and collaborate on
refining outputs collectively within teams or communities devoted to exploring
LLM’s
Document Your Experiments: Keep tune of what works and what doens’t .Taking
notes and documentation results will help you refine your workflow, troubleshoot
problems, and improve.
What’s in store for 2024?
LLMs transforming customer experience and self service
Customer experience (CX) is the cornerstone of business success. In an era where customers
demand personalized interactions and instant solutions, large language models have emerged as
game-changers. By analyzing vast amounts of customer data, understanding language nuances,
organizations can provide responses that feel tailor-made for each individual.

Why pick customer care as your AI use case
1. Personalized interactions: Large language models have the capability to analyze
customer data, including past interactions, purchase history, and preferences, to
generate responses that resonate with each customer. This personalization goes
beyond using the customer’s name in an email – it involves understanding the
customer’s intent, sentiment, and context to deliver relevant and meaningful
interactions. For instance, a customer reaching out to a retail company’s chatbot
about a recent purchase can receive personalized recommendations for
complementary products, enhancing their shopping experience.
2. Natural language understanding: Large language models can comprehend and
respond to natural language queries, eliminating the need for customers to adapt
their language to the system. This level of understanding fosters smoother
conversations, reduces customer frustration, and creates a more positive impression
of the brand’s commitment to customer service.
3. LLMs save time and resources: Time is money, and contact centers are always on
the lookout for ways to optimize processes and minimize resource expenditure.
Large language models come to the rescue by automating tasks that once required
significant human involvement.
4. Automated customer support: Traditionally, customer support involved large
teams of agents fielding inquiries around the clock. With large language models,
businesses can deploy AI-powered chatbots that handle routine queries, provide
instant responses, and even escalate complex issues to human agents when
necessary. This not only reduces the workload on human agents but also ensures
customers receive prompt assistance, irrespective of the time of day.
5. Streamlining workflows: Large language models can be integrated into various
business tools and software, acting as intelligent assistants that help employees
navigate complex tasks more efficiently. Whether it’s drafting emails, generating
reports, or analyzing data, these models can learn from existing patterns and
produce outputs that align with established company standards.
However there are potential pitfalls
It is crucial to know that there might be certain drawbacks
1. Data Bias: One disadvantage is the problem of bias. Since LLMs study from
massive amounts of text statistics generated by way of humans, they can
inadvertently inherit biases present in those records. In this manner if the schooling
facts carry biased language or perspectives, the model might generate outputs that
perpetuate the ones biases.
2. Generating Misinformation: Another problem while the use of LLMs is their
tendency to supply workable-sounding but wrong information. LLMs do not
inherently fact-check the information they generate. If a prompt instructs the model
to provide information without fact-checking, it may generate content that is
factually incorrect or misleading.
3. Computational Resources: Training and deploying large language models require
substantial computational resources, including powerful GPUs or TPUs. This can lead
to high infrastructure costs and limited accessibility for smaller organizations or
individual developers.
4. Energy Consumption: Training large language models consumes a significant
amount of energy. This has raised concerns about the environmental impact of deep
learning models, especially when they are trained on large-scale data centers.
5. Privacy: Privacy is a significant challenge while running with massive language
models. Generating awesome textual content regularly entails inputting sensitive
statistics into those systems, elevating questions on statistics safety and
confidentiality.
Despite these challenges, researchers and organizations are actively working on ways we
can incorporate them into everyday lives

Source: National institute for Standards and technology
Future Outlook for LLM’
The benefits are undeniable - from producing refined content in a fragment of the time to
automating mundane obligations, LLM’s truly hold the key to increase productivity by 5X
However, it's crucial to note that there will also be a few drawbacks. One such issue is bias
within those models because of biased training records. It's important for users to remain
vigilant and attempt closer to moral AI practices that promote equity and inclusivity.
As thrilling as this all sounds, it is vital no longer just to depend completely on large
language models however additionally integrate them with human creativity and essential
questioning abilities. After all, no matter how state-of-the-art an algorithm becomes, it still
lacks human instinct and empathy.
So, Incorporate them into your workflow strategically; leverage their power wisely; But
don't forget: at its middle lies human ingenuity – something that no synthetic intelligence
can absolutely replace.
In this era where in technological improvements are reshaping our world each passing day
allow us to harness these innovations responsibly at the same time as striving for a
harmonious stability among man and system.